Deep Learning: Generative Models
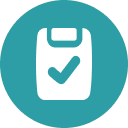
Assessment
•
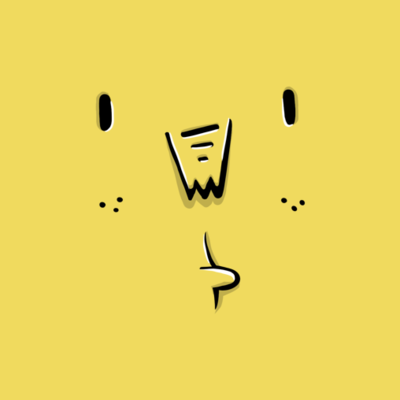
Josiah Wang
•
Mathematics, Science, Computers
•
University
•
35 plays
•
Hard
Student preview
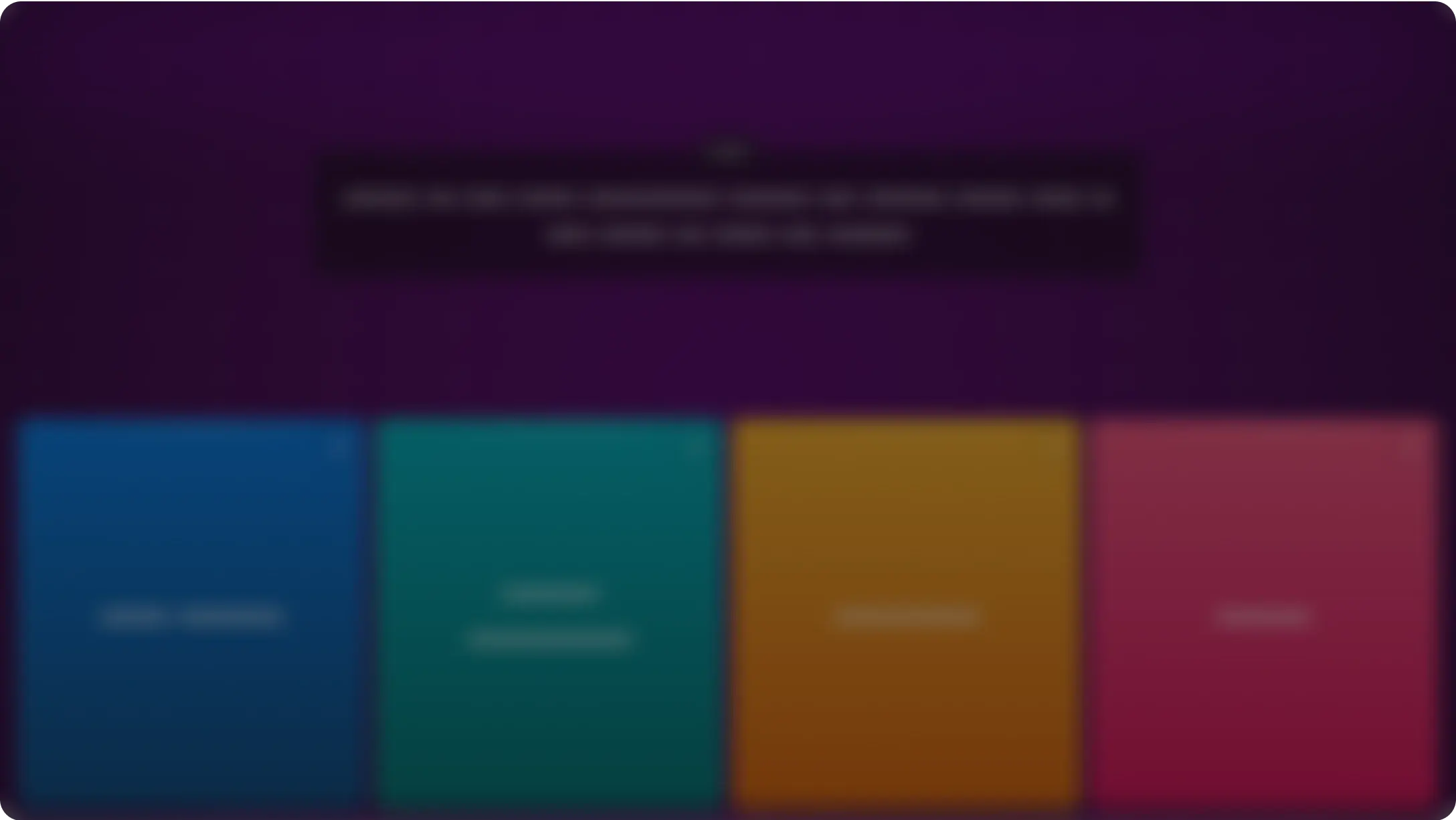
10 questions
Show all answers
1.
MULTIPLE SELECT
15 mins • 1 pt
Which of the following statements justify the Maximum Likelihood approach ?
Answer explanation
Definition of the likelihood function as “the likelihood of the model parameters that explains the generation of the data”, so MLE corresponds to finding the “best explanation”.
With regards to both the KL options - refer to the definition of KL.
Maximum Likelihood minimises the reconstruction error only if the model likelihood itself describes a reconstruction process - think cross-entropy loss.
2.
MULTIPLE SELECT
15 mins • 1 pt
Which of the following statements, when combined together, explain why we cannot train VAEs using Maximum likelihood Estimation?
Answer explanation
MLE requires the evaluation of
to marginalise out the latent variable. This is intractable due to the nature of p(x|z)The option 'The latent variable is continuous' is not true if picked alone -- consider probabilistic PCA where the latent variable is Gaussian and the “decoder” is linear.
Regarding the option about there being too many datapoints, this is about intractability due to large-scale data, not due to the intractability of marginal likelihood on each datapoint.
3.
MULTIPLE SELECT
15 mins • 1 pt
Which of the following statements are true for the VAE objective?
Answer explanation
The gap between the VAE and Maximum Likelihood objective is not KL[p(z)||q(z|x)] -- check definitions.
The KL term acts as a reguraliser when the prior is fixed with no learnable parameters. If prior is learnable, the prior can be learned towards the q distribution so the regularisation effect is unclear.
The optimum of the VAE == the MLE optimum only if q is the true posterior, so the correctness of this statement depends on the form of q.
4.
MULTIPLE SELECT
15 mins • 1 pt
In the famous “Chinese room” turing test example, a man will be sitting inside a room doing English-to-Chinese translation, and the other volunteers outside the room will be asked to guess, based on the English-to-Chinese translation results, whether the man in the room understands Chinese or not. You are one of the volunteers. You know the man in the room is English so you assume a priori he does not understand Chinese with probability 0.8. Now given the translation result is correct, how would you guess whether he understands Chinese or not?
Answer explanation
The goal of this question is to guide students to think about Bayes’ optimal classifier. This requires information about p(translation is correct | the man only speaks English) and p(translation is correct | the man speaks both English and Chinese).
5.
MULTIPLE CHOICE
15 mins • 1 pt
Which best represents the reparameterisation trick?
Answer explanation
You cannot backprop through a stochastic node. The reparamaterisation trick allows you to emulate sampling from a distribution however keeping the main computational graph ( and ) deterministic and so differentiable.
6.
MULTIPLE SELECT
15 mins • 1 pt
Which of the following statements are true for the encoder in a Variational Autoencoder.
Answer explanation
VAEs are latent variable models, in that they use a latent variable, z to describe the generation process. Now in order to calculate p_model(x), rather than having to sample all values of z (which results in an intractable problem) the encoder is introduced as an approximate posterior to narrow down the latent space and suggest likely latent codes given x.
7.
MULTIPLE CHOICE
15 mins • 1 pt
Heuristically which of the two plots is the best loss for the Generator in a Generative Adversarial Network?
Answer explanation
This is heuristically motivated. Maximising the probability that the discriminator makes a mistake rather than minimising the probability that the discriminator is correct results in the derivatives of the generator’s loss function with respect to the discriminators logits to remain large even when the discriminator easily rejects the generators samples.
8.
MULTIPLE CHOICE
15 mins • 1 pt
Mode collapse is when...
Answer explanation
One of the main failure modes for GANs is for the generator to collapse to a parameter setting where it always emits the same point. When collapse to a single mode is imminent, the gradient of the discriminator may point in similar directions for many similar points. Because the discriminator processes each example independently, there is no coordination between its gradients, and thus no mechanism to tell the outputs of the generator to become more dissimilar to each other. Instead, all outputs race toward a single point that the discriminator currently believes is highly realistic. After collapse has occurred, the discriminator learns that this single point comes from the generator, but gradient descent is unable to separate the identical outputs. The gradients of the discriminator 2 then push the single point produced by the generator around space forever.
9.
MULTIPLE CHOICE
15 mins • 1 pt
This figure details the different output signals generated from an optimal discriminator for an original GAN and a Wasserstein GAN. The blue and green dots represent the 1D real and fake data instances. Match the colour line with the type of GAN.
Answer explanation
The Wasserstein distance or Earth-Mover distance re-frames the comparison to (intuitively) what is the cost of optimally moving all the probability mass (or earth) from one distribution to the other. Key is that the Wasserstein metric provides continuous and useful gradient signals no matter what the difference or distance between the two distributions is. This is extremely helpful for GAN training as even if the discriminator is easily distinguishing between real and fake images the generator can still learn. The Wasserstein discriminator provides useful gradients for all areas of the one dimensional explorable space, where as the standard GAN gradients quickly vanish/are not useful for the majority of the search-able space
10.
MULTIPLE SELECT
15 mins • 1 pt
Which of the following statements are true about Beta-VAEs? (note: beta is the coefficient of the KL term)
Answer explanation
The larger beta is, the more weight is placed on the approximate posterior matching the sampling prior.
Explore all questions with a free account
Similar Resources on Quizizz
Proofs, Postulates, Theorems
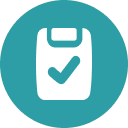
•
10th Grade - University
Intro to Proofs Geometry
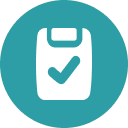
•
9th Grade - University
Segments Angles
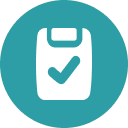
•
11th Grade - University
Squares Rectangles
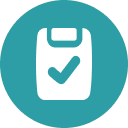
•
5th Grade - University
[IMV] Future Edge, Generative AI
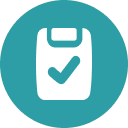
•
University
Relationships in Triangles
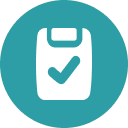
•
10th Grade - University
Measurement Metric Length Mass and Capacity
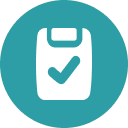
•
4th Grade - University
Radiações
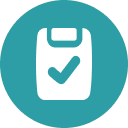
•
University
Popular Resources on Quizizz
STAAR reading review
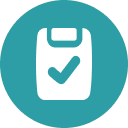
•
4th - 5th Grade
7th STAAR Reading Review
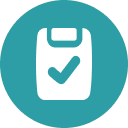
•
7th Grade
STAAR Reading Review
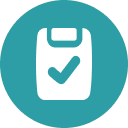
•
4th - 7th Grade
STAAR reading vocabulary
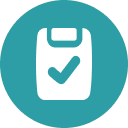
•
4th - 5th Grade
STAAR Reading Review
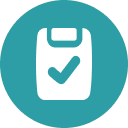
•
3rd - 5th Grade
Reading STAAR Review
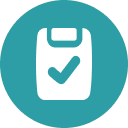
•
4th Grade
7th grade STAAR Reading Review
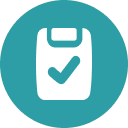
•
7th Grade
Revising and Editing
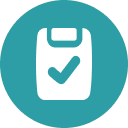
•
4th Grade